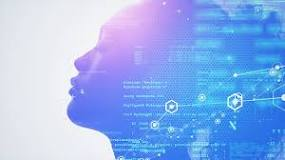
Duration
21 hours (usually 3 days including breaks)
Requirements
There are no specific requirements needed to attend this course.
Overview
Introduction:
Deep learning is becoming a principal component of future product design that wants to incorporate artificial intelligence at the heart of their models. Within the next 5 to 10 years, deep learning development tools, libraries, and languages will become standard components of every software development toolkit. So far Google, Sales Force, Facebook, Amazon have been successfully using deep learning AI to boost their business. Applications ranged from automatic machine translation, image analytics, video analytics, motion analytics, generating targeted advertisement and many more.
This coursework is aimed for those organizations who want to incorporate Deep Learning as very important part of their product or service strategy. Below is the outline of the deep learning course which we can customize for different levels of employees/stakeholders in an organization.
Target Audience:
( Depending on target audience, course materials will be customized)
Executives
A general overview of AI and how it fits into corporate strategy, with breakout sessions on strategic planning, technology roadmaps, and resource allocation to ensure maximum value.
Project Managers
How to plan out an AI project, including data gathering and evaluation, data cleanup and verification, development of a proof-of-concept model, integration into business processes, and delivery across the organization.
Developers
In-depth technical trainings, with focus on neural networks and deep learning, image and video analytics (CNNs), sound and text analytics (NLP), and bringing AI into existing applications.
Salespersons
A general overview of AI and how it can satisfy customer needs, value propositions for various products and services, and how to allay fears and promote the benefits of AI.
Course Outline
Day-1:
Basic Machine Learning
Module-1
Introduction:
- Exercise – Installing Python and NN Libraries
- Why machine learning?
- Brief history of machine learning
- The rise of deep learning
- Basic concepts in machine learning
- Visualizing a classification problem
- Decision boundaries and decision regions
- iPython notebooks
Module-2
- Exercise – Decision Regions
- The artificial neuron
- The neural network, forward propagation and network layers
- Activation functions
- Exercise – Activation Functions
- Backpropagation of error
- Underfitting and overfitting
- Interpolation and smoothing
- Extrapolation and data abstraction
- Generalization in machine learning
Module-3
- Exercise – Underfitting and Overfitting
- Training, testing, and validation sets
- Data bias and the negative example problem
- Bias/variance tradeoff
- Exercise – Datasets and Bias
Module-4
- Overview of NN parameters and hyperparameters
- Logistic regression problems
- Cost functions
- Example – Regression
- Classical machine learning vs. deep learning
- Conclusion
Day-2 : Convolutional Neural Networks (CNN)
Module-5
- Introduction to CNN
- What are CNNs?
- Computer vision
- CNNs in everyday life
- Images – pixels, quantization of color & space, RGB
- Convolution equations and physical meaning, continuous vs. discrete
- Exercise – 1D Convolution
Module-6
- Theoretical basis for filtering
- Signal as sum of sinusoids
- Frequency spectrum
- Bandpass filters
- Exercise – Frequency Filtering
- 2D convolutional filters
- Padding and stride length
- Filter as bandpass
- Filter as template matching
- Exercise – Edge Detection
- Gabor filters for localized frequency analysis
- Exercise – Gabor Filters as Layer 1 Maps
Module-7
- CNN architecture
- Convolutional layers
- Max pooling layers
- Downsampling layers
- Recursive data abstraction
- Example of recursive abstraction
Module-8
- Exercise – Basic CNN Usage
- ImageNet dataset and the VGG-16 model
- Visualization of feature maps
- Visualization of feature meanings
- Exercise – Feature Maps and Feature Meanings
Day-3 : Sequence Model
Module-9
- What are sequence models?
- Why sequence models?
- Language modeling use case
- Sequences in time vs. sequences in space
Module-10
- RNNs
- Recurrent architecture
- Backpropagation through time
- Vanishing gradients
- GRU
- LSTM
- Deep RNN
- Bidirectional RNN
- Exercise – Unidirectional vs. Bidirectional RNN
- Sampling sequences
- Sequence output prediction
- Exercise – Sequence Output Prediction
- RNNs on simple time varying signals
- Exercise – Basic Waveform Detection
Module-11
- Natural Language Processing (NLP)
- Word embeddings
- Word vectors: word2vec
- Word vectors: GloVe
- Knowledge transfer and word embeddings
- Sentiment analysis
- Exercise – Sentiment Analysis
Module-12
- Quantifying and removing bias
- Exercise – Removing Bias
- Audio data
- Beam search
- Attention model
- Speech recognition
- Trigger word Detection
- Exercise – Speech Recognition