Since the early 1950s, machine learning (ML) has developed from playing a simple game of checkers to extremely advanced algorithms that help humans with everything from predicting the remaining useful life (RUL) of jet engines to the production of self-driving cars. In recent years, ML has allowed companies to automatically detect damage to powerlines, predict the best time to buy a product online, and even build effective fraud detection systems, all adding to the convenience and safety of our lives. Capitalizing on these technologies and incorporating them into existing Altair products allows engineers to work in a smarter way, speeding up design and production time.
There are a multitude of techniques when using ML that offer different solutions for different problems, and when considering how ML can be utilized in the engineering world it is important to understand the different approaches and methods. In its simplest organization, the two main categories are supervised and unsupervised, each with its own objectives and uses. Under the umbrella of these methods, a range of applications can be explored and optimized to provide faster workflow, optimized design, and more accurate predictions.
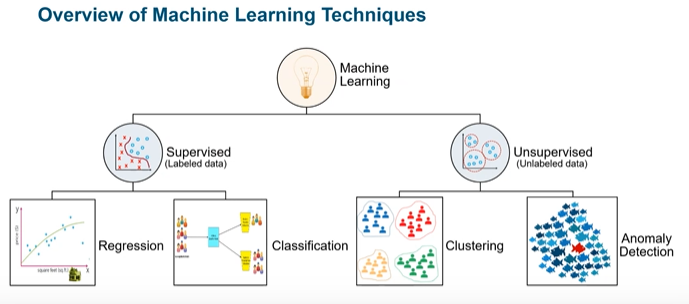
Although incorporating this collection of technology is relatively new in the field of engineering, Altair has started to make leaps forward in this space to provide its users with the tools they need to make a difference. Take CAD tasks and 3D design for example. ML can act as a powerful tool to aid in this discipline, ultimately leading to optimized methods of manufacturing and more accurate simulations.
When building a CAE/CAD model, being able to search for similar shapes can be very useful. By selecting a part, it is now possible to search for similar shapes in Altair HyperWorks™, saving the user time and effort. Going one step further, part clustering will take all parts included and cluster them with respect to their shape similarity, allowing the user to view all clusters within a build.
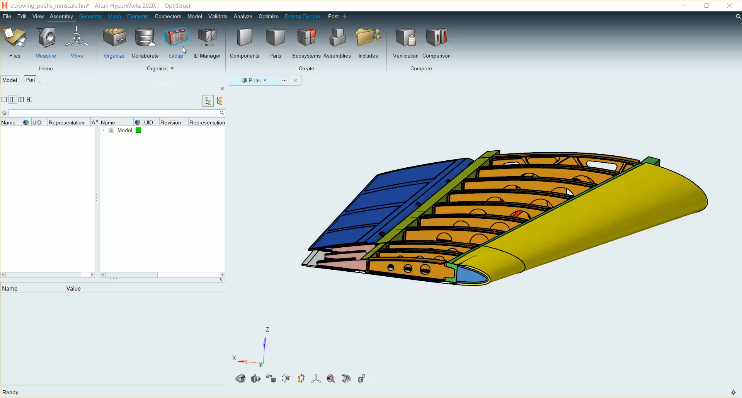
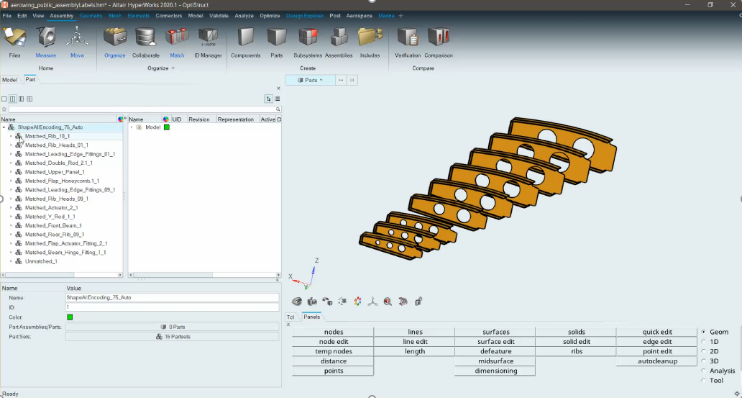
ML can also help with the accuracy of a simulation. Incorporating ML into Altair AcuSolve™ has led to improved aerodynamics predictions by implementing a physics-informed data-driven ML model. This produces a more accurate prediction of flow separation when studying aerodynamic fluid flow in conjunction with a deep neural network (DNN).
This process is achieved by appending a correction term to the equations governing the fluid flow and running several simulations with adjoint optimization to obtain training data. The key learning features are then identified in the generated training data and used to train and test the DNN models for the correction term.
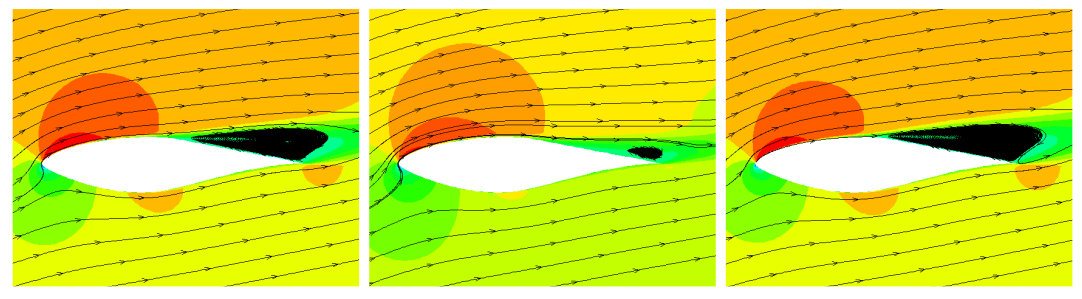
A design of experiments (DOE) methodology allows engineers to create variables, responses, and goals to obtain the best design results possible. When used in conjunction with a conventional ML prediction model, this leads to predicted KPIs. More recently, advances in ML methods and engineering software have made it possible to make physics predictions leading to accurate contour plots, represented visually in real time.
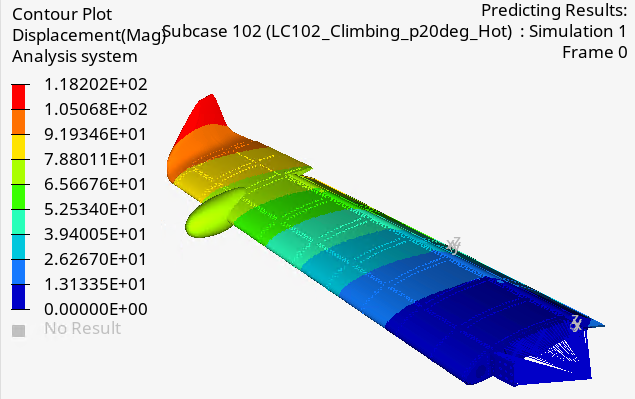
By leveraging field predictive ML models engineers can explore more options without the use of a solver when designing different components and parts, saving time and resources. This ultimately produces higher quality results that can then be used to make more informed decisions throughout the design process.
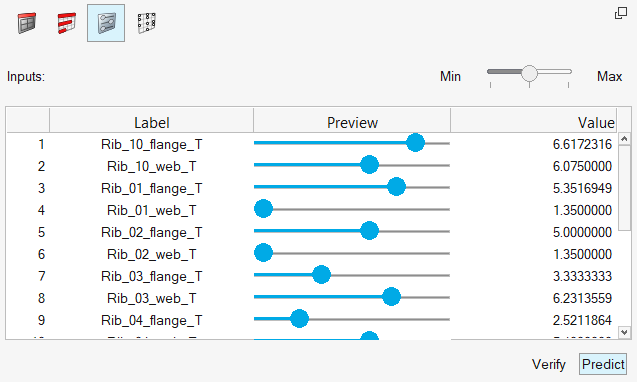
In optimization, it is sometimes desirable but not possible to define constraints that fully reflect an expert’s requirements. This may lead to a design that does not function as intended. ML enables the user to set up subjective constraints to ensure a design that has been trained to replicate the expert’s opinion. In the automotive industry for example, this can be a huge advantage.
In a project with one of Altair’s major customers, ML methods were successfully employed to create a concept design for a reinforced bracket subjected to crash loads. The design space was sampled to avoid any folding modes after the crash event, with the modes then clustered to label them more easily.
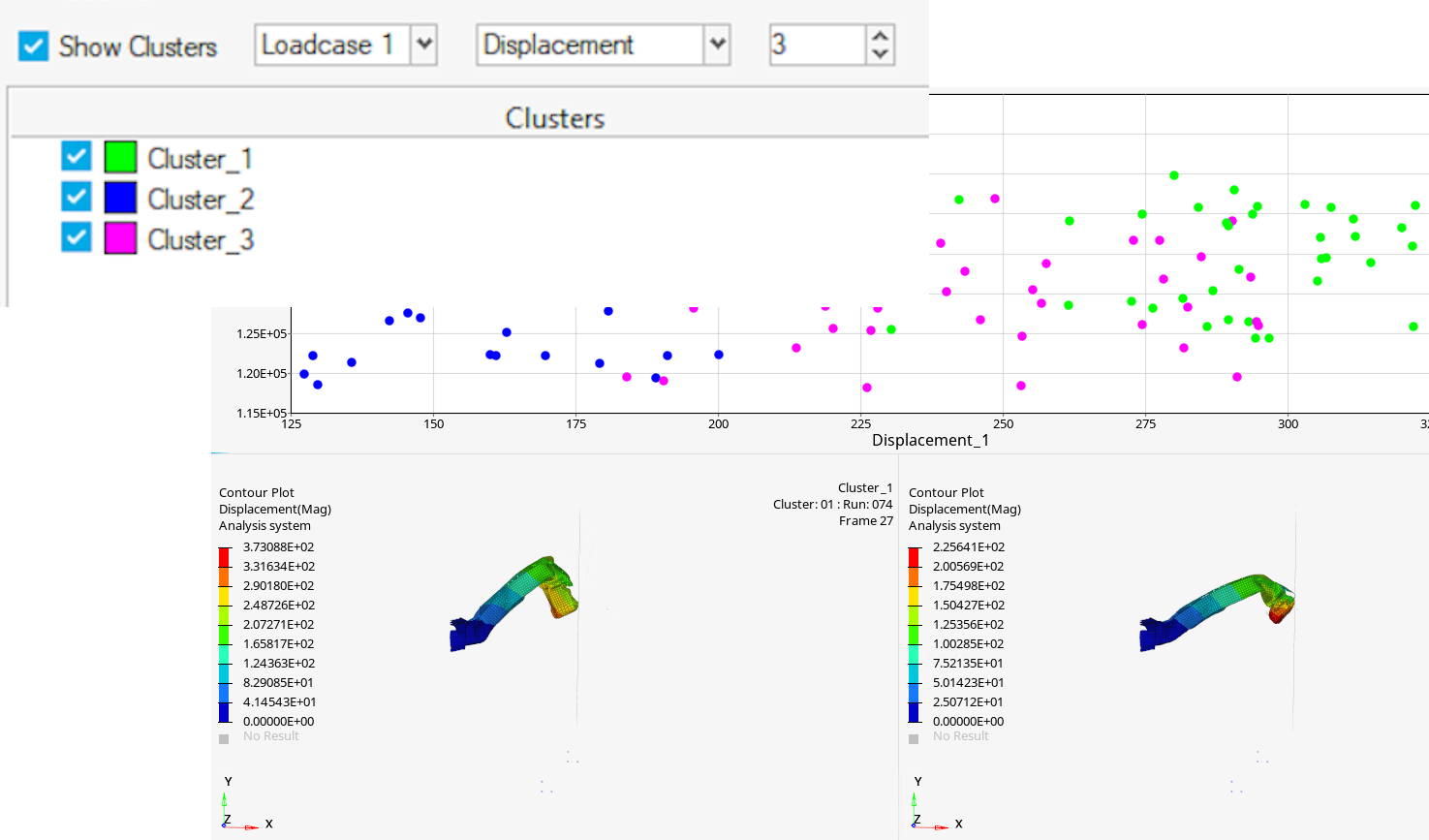
These designs could then be classified based on the desired shape and used to teach a machine learning model. By doing this, it is possible to incorporate expert preferences in an optimization, leading to faster design cycles and improved design.
Altair DesignAI enables teams to start enhancing product design by using existing design and simulation data, and scaling internal expertise. Altair DesignAI is a cloud native solution accessible on Altair One that helps organizations save time and money in the product development process. Altair One allows users to quickly find and download Altair and partner software to solve your giving collaborative access to simulation and data analytics technology plus scalable HPC and cloud resources, all in one place.
These advances to Altair solutions and machine learning techniques make it possible to work in a more effective way, producing results faster. By combining physics-based, simulation-driven design and machine learning-based AI-driven design, users are able to efficiently identify high-potential designs and reject low-potential designs earlier in development cycles.
Designed for people with different skill sets, our desktop-based predictive analytics and ML solutions helps users to quickly generate actionable insights from data. Quickly build out predictive and prescriptive models that easily explain and quantify insight found in your data. Apply and share that insight by deploying models natively or exporting them to common business intelligence (BI) tools. Data scientists rely on Altair to efficiently build powerful and insightful predictive models to make better business decisions.