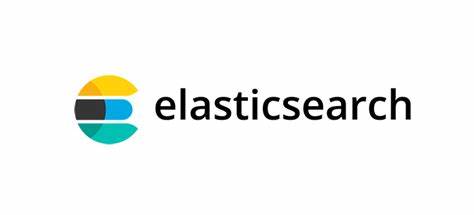
Introduction to Elasticsearch
Writing Search Queries
Performing Text Analysis
Defining Mappings
Expanding Your Searches
The Distributed Model
Manipulating Search Results
Performing Aggregations
Handling Data Relationships
Introduction to Elasticsearch
Writing Search Queries
Performing Text Analysis
Defining Mappings
Expanding Your Searches
The Distributed Model
Manipulating Search Results
Performing Aggregations
Handling Data Relationships
The Elasticsearch Platform natively integrates powerful machine learning and AI into solutions — helping you build applications users crave and get work done faster.
Get immediate value from machine learning with domain-specific use cases built right into Elasticsearch. With observability, search, and security solutions, DevOps engineers, SREs, and security analysts can get started right away. No prior experience with machine learning required.
Teams can automate anomaly detection and root cause analysis, reducing mean time to repair (MTTR). In addition, built-in capabilities such as natural language processing (NLP) and vector search help teams implement search experiences that are easier for end users.
Use Elastic machine learning to:
Accelerate problem detection and resolution with automated anomaly detection, correlations, and other AIOps capabilities built into Elastic Observability. DevOps and SRE teams can identify unusually slow response times directly from the APM service map. You can apply machine learning without having to configure models.
Machine learning powers threat detection in Elastic Security. You can reduce mean time to resolution (MTTR) by automatically identifying unusual activity in the SIEM app. For threats that are difficult to identify, supervised models can disambiguate suspicious from benign activity, for example for living off the land attacks or domain generated algorithms.
With Elasticsearch, you can build search-powered applications using natively run vector search and NLP to achieve superior search relevance, performance, and personalization. Reference the models while configuring the ingestion pipeline, as shown on the right.
Apply Elastic machine learning to your data to:
To apply Elastic machine learning, you don’t need to have a data science team or design a system architecture. Our machine learning capabilities allow you to quickly get started! There’s no need to move data to a third-party framework for model training.
For those use cases that require custom models and optimized performance, our tools let you adjust parameters and import optimized models from the PyTorch framework.
Elastic’s out-of-the-box integrations make data ingestion and connecting to other data sources easy. Once your data is in Elasticsearch, you can visualize and gain initial insights in minutes.
Elastic’s open, common data model, Elastic Common Schema (ECS), gives you the flexibility to collect, store, and visualize any data. This includes metrics, logs, traces, content, and events from your apps and infrastructure. To start, choose your ingest method. Options include Elastic Agent, web crawler, data connectors, and APIs, and we have native integrations with all major cloud providers. Once your data is in Elastic, built-in tools — like Data Visualizer — help you identify fields in your data that would pair well with machine learning.
No experience applying machine learning? Apply the preconfigured models for observability and security. If those don’t work well enough on your data, in-tool wizards guide you through the few steps needed to configure custom anomaly detection and train supervised learning.
Unsupervised machine learning with Elastic helps you find patterns in your data. Use time series modeling to detect anomalies in single or multiple time series, population data, and forecast trends based on historical data.
You can also detect anomalies in logs by grouping messages, and uncover root causes by reviewing anomaly influencers or fields correlated with deviations from baselines.
To categorize your data and make predictions, train classification or regression models using data frame analytics in Elastic. Supervised models get you closer to the root cause of issues and can drive intelligent decisions in your applications.
You can use continuous index to convert application logs index into a user-centric activity view and build a fraud detection model using classification. Then you can apply your models to your incoming data at ingest, all without ever leaving Elastic.
Vector semantic search lets your users find what they mean, instead of being limited to keywords. They can search through textual data, images, and other unstructured data.
With Elastic machine learning, you can implement semantic search to make digital experiences more intuitive and results more relevant. Examples include:
To get started, Elastic lets you import pre-trained BERT-like PyTorch models from hubs, like Huggingface.co, or the CLIP model from OpenAI. Learn more about implementing image similarity with Elastic.